Technology Adoption Lifecycle & "Crossing the Chasm" with AI in 2024
How come customers and product are at a disconnect?
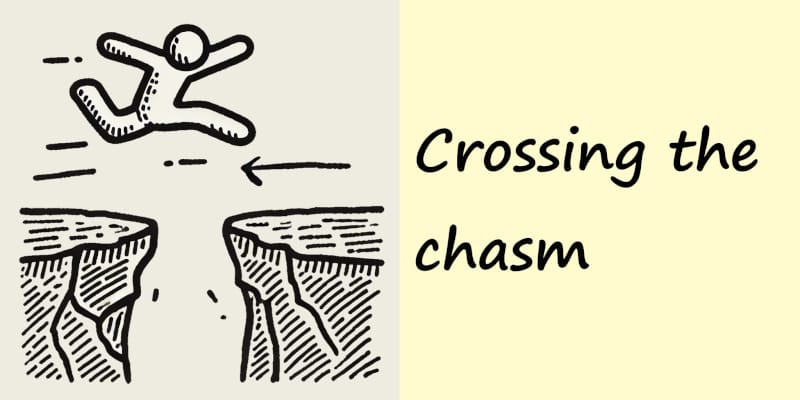
What's Going On with AI in Our Products and Why Are Customers Struggling to Use Them While Tech Companies Forge Ahead?
Back at the end of the 1980s, Warren Schirtzinger & Lee R. James of Regis McKenna Inc. (RMI) developed the Diffusion of Innovations model into what we now recognize in marketing circles as the Technology Adoption Lifecycle or The 5 Customer Segments of Technology Adoption. This model illustrates the acceptance of a new product in terms of the customers it attracts over its market lifespan.
They also introduced the concept of the chasm in the curve and later published the popular book Crossing the Chasm. In the Crossing the Chasm Summary, they state that the analysis seeks to "explain how, why, and at what rate new ideas and technologies are adopted".
What they observed is that technology products do not follow the same adoption profile over time as non-technical products:
"James and Schirtzinger identified the point of greatest difficulty in the development of a high-tech market as a transition from an early market dominated by early adopters to a mainstream market dominated by a large group of customers who are realistic and practical."
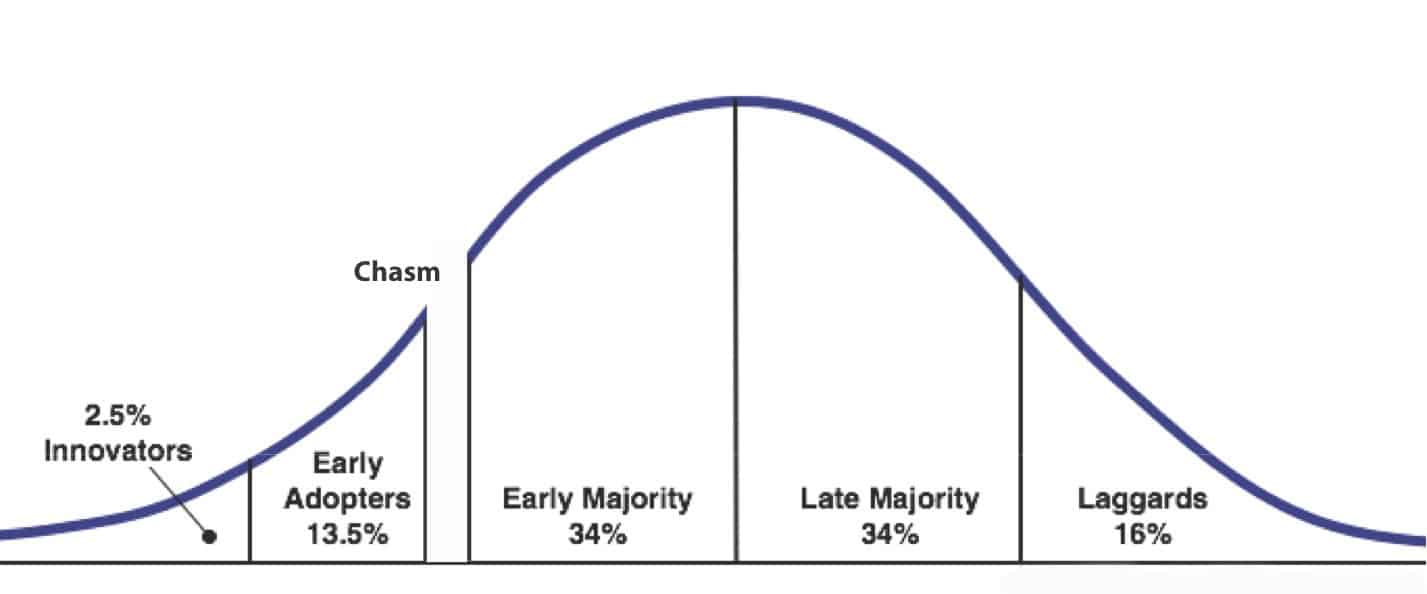
This point of difficulty became known as "the marketing chasm." Essentially, it's a disconnect that arises when the focus shifts from being technology-driven to human-driven. This is key to understanding what we are seeing with AI today: we are trying to cross the chasm.
Early adopters are more comfortable with intangibles than the Early Majority. Developers have embraced AI tooling, while mainstream users struggle with the inherent unpredictability of current AI LLMs. These users seek bulletproof solutions—something the industry is still addressing with guardrails and verification stages in generative AI pipelines. The Early Majority wants predictable, reliable, and accurate results from AI, which is fundamentally at odds with the probabilistic math that drives AI.
Today, Jeremy Vyska published a post sharing a familiar story about AI, customers, and the real world. In his post, Sometimes Cutting Edge is Bleeding Edge, he described how a customer was using the AI helper "CoPilot" in the ERP software Business Central to achieve specific tasks with accounting dimensions (to the layperson, dimensions are the way the ERP system routes money internally).
The problem with AI LLMs is that they are eager to please, much like a small child wanting to answer questions even if they don't know the answer. This leads to a phenomenon known as hallucinations, where totally fictitious output is generated. This output is shaped by the way the question is asked because the LLM wants to satisfy the user by giving them what they expect to hear—a machine version of a people-pleaser. The LLM will also respond with such confidence that we feel compelled to accept its answers as factual, even when they are not grounded in reality.
The Challenge of Adoption After the Chasm
The Early Majority, unlike early adopters, are not as willing to tolerate beta software and uncertainty. This creates the disconnect we are now experiencing with AI in everyday office software, including ERP systems.
Learning to Use Google in the 1990s
The quality of AI output depends heavily on how well the prompts are crafted. Prompt engineering has become a specialized skill because better prompts can significantly mitigate hallucinations.
Think back to how we had to learn to use Google. Today, searching on Google feels natural, but in the 1990s, we had to develop search as a skill. I know from personal experience that my own mother found learning to use Google a challenge. Similarly, using AI effectively at this stage requires well-crafted prompts.
The Need for Verification
A verification step is essential for applications requiring high accuracy, such as medical notes, compared to more creative uses like poetry, where deviation from fact is acceptable. Modern AI solutions are incorporating verification steps to double-check the accuracy of generated content. Techniques like RAG (Retrieval-Augmented Generation) are also being used to improve results, but that's a more technical discussion for another time.
Forcing AI Over the Chasm
Now we reach the main point. The tech industry is in a gold rush to get AI in front of customers, driven by the fear of being left behind if they don’t have "AI" as a feature in their product lineup. The industry is pushing AI over the chasm to the Early Majority, but I fear these users are not ready for it. They expect mature, reliable products that current AI simply isn't yet. While these AI solutions are powerful in the right, tolerant hands (early adopters), we are still in the early days of AI development.
As Jeremy put it, "new technology is just that – new". From the perspective of software companies, they are trying to rocket the train over the chasm, action-movie style, to drive adoption. But this rush makes for a bumpy ride. Supporting partners of AI solutions will face a prolonged period of managing customer expectations while these products continue to innovate and mature, eventually becoming more palatable to the Early Majority and Late Majority.
My hand drawn illustration, below, is how [this Forbes article AI that can invent AI is coming, buckle up],(https://www.forbes.com/sites/robtoews/2024/11/03/ai-that-can-invent-ai-is-coming-buckle-up/) predicts super AI growth, due to AI inventing better AI. Together with quantum computing that is now on the horizon, AI will become much more capable, we are just looking up at the cliff from below...:
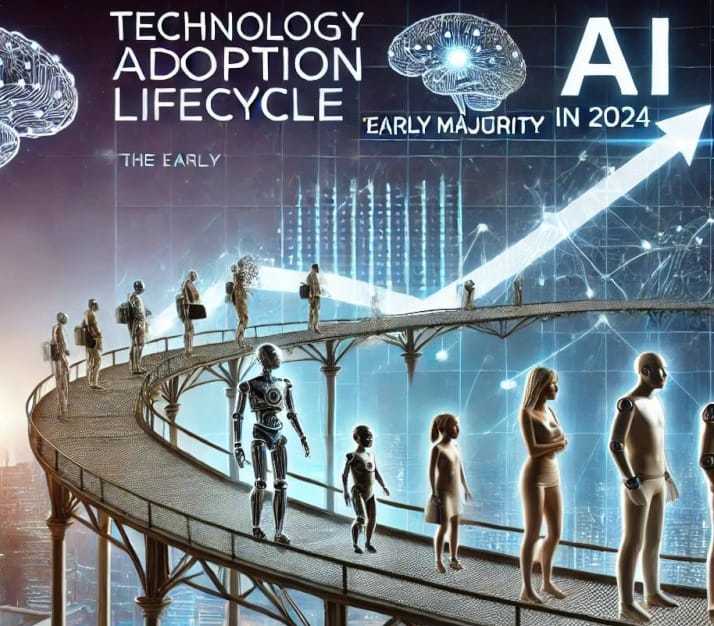