Riding the "S" curve of AI growth
Academic and business growth of AI and recent news on Amazon giving academics more compute.
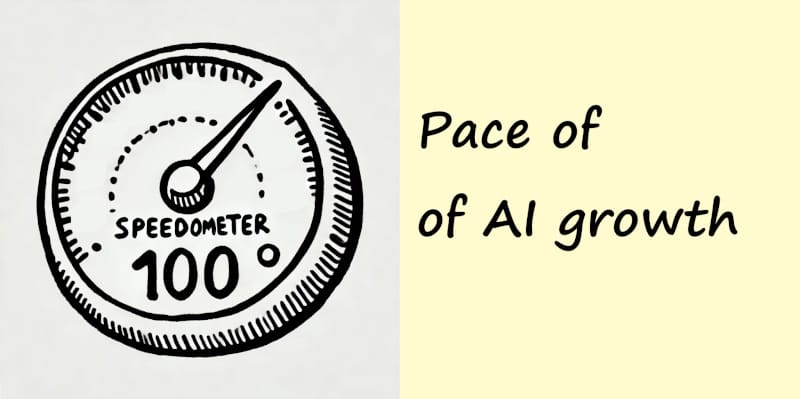
AI is experiencing "exponential growth", or maybe it is an "S" curve?
Although it is not exponential, it is nonetheless miraculous progress we have seen recently with AI, particularly in the area of Large Language Models and generative AI. There is not a new generation Claude, ChatGPT, and Gemini every month, then week, then day, so it feels like it isn't really exponential in nature.
Large Language Models are an area of AI/Machine Learning that has seen particularly remarkable breakthroughs recently. Other areas are also evolving and will soon be working together with LLMs to get closer to the ultimate goal of Artificial General Intelligence (AGI).
When I came to research this blog post, it struck me that there are two very different types of AI progress:
AI Academic research
AI in real business
The pace of development in these two areas is measured by different metrics and can be conflated when the media talks about advances in AI. I would argue the important metrics of progress are very different between the two.
I also got me into thinking about the difference of what is happening in universities vs big tech.
The Uneven Playing Field Between Academia and Big Tech
Universities have historically been hubs of groundbreaking discoveries, but in the AI era, the scale of resources required for meaningful progress seems to tilt the field in favour of those who already hold computational power. See this lovely paper that highlights some of that frustration from an academic: In this paper.
Quote from that paper referencing Google DeepMind, OpenAI, and Meta AI:
On the one hand: it is very impressive. Good on you for pushing AI forward. On the other hand: how could we possibly keep up? As an AI academic, leading a lab with a few PhD students and (if you’re lucky) some postdoctoral fellows, perhaps with a few dozen Graphics Processing Units (GPUs) in your lab, this kind of research is simply not possible to do.
When you have been working on a research topic for a while and, say, DeepMind or OpenAI decides to work on the same thing, you will likely feel the same way as the owner of a small-town general store feels when Walmart sets up shop next door. Which is sad, because we want to believe in research as an open and collaborative endeavour where everybody gets their contribution recognised, don’t we?
AI has economic and sociological impacts that attract a lot of funding and prestige to drive research, but access to big compute neural processing units has been limited. Breakthroughs in AI will continue to be made with better algorithms coming out of continued developments in machine learning. Big Tech is starting to help out by giving universities access to AI cores. I found this recent announcement particularly heartening: Amazon Puts $110M Into Academic Generative AI Research. Here, Amazon Web Services (AWS) has created a computer cluster where researchers can make reservations to access up to 40,000 Trainium chips. Let's hope this initiative is followed by other leaders in the industry too. Academic research is not bound by the same commercial goals as corporate research, so it is free to explore things that are not a priority for the big corporations. Let's hope some good for society comes out of these gestures.
Uni vs Big Corp
-
Corporations primarily focus on applied research geared toward profit-driven outcomes, such as developing large language models, recommendation systems, and algorithms to enhance advertising.
-
Universities tend to emphasise theoretical research, diving into novel algorithms, interdisciplinary uses of AI, and ethical questions.
-
Corporations invest heavily in scaling up AI, optimising for efficiency in production systems.
-
Universities, with fewer resources, take on fundamental challenges like innovating new learning paradigms, creating AI solutions that require less data, and improving the interpretability and robustness of AI models.
-
Corporations often address bias and fairness as part of brand protection efforts but are inclined to prioritise solutions that support their business objectives.
-
University researchers, meanwhile, are more likely to examine the societal implications of AI, including issues of equity, access, and how automation affects jobs and communities.
-
Corporations generally approach legal and ethical concerns with compliance in mind, ensuring their products align with regulations while preserving a competitive edge.
-
Universities, however, engage more deeply in policy-shaping research. Academics study governance frameworks and the ethical implications of AI, often taking a critical view of corporate practices.
-
Corporate research may be constrained by profit motives, sometimes leading to censorship.
-
University researchers have greater freedom to pursue out-of-the-box thinking or long-term ideas, although they often face limitations due to funding constraints.
Universities also emphasise cross-disciplinary research, often integrating AI with philosophy, sociology, and ethics.
In my view, AI progress in business is rooted in more tangible, often commercial-oriented metrics than the metrics used to measure progress in the more theoretical world of academia.
Measuring AI Progress in Business
I created this list of what I see as important:
- Business Adoption of AI: The extent to which AI tools and systems are integrated into workflows across industries.
- Cost of AI Operations: The expense of deploying, running, and maintaining AI systems, including infrastructure, hardware, and personnel.
- Performance: The quality of AI outputs, including accuracy, reliability, and relevance to business goals.
- Overall Efficiency: The impact of AI on reducing operational costs, improving turnaround times, or increasing productivity.
- Scalability: How effectively AI solutions can grow with the business, adapting to higher demands or broader applications.
Scalability is currently the area receiving the most focus from what I see out there.
The AI gold rush boosts data centre demand
There is a new wave of data centres being built to meet the demand for compute in the AI revolution. These data centres need plenty of power. I have personally been wondering if the lack of energy supply will stunt this "exponential" growth of AI, and how the price of energy, applying supply and demand theory, will affect this.
However, innovation is at work. Advances in efficient chips, Neural Processing Units (NPUs), and dedicated chips for doing efficient AI maths are emerging. Then we have Google looking at nuclear reactors (eek!) to power AI needs: Google turns to nuclear to power AI data centres.
On adoption, while startups embrace AI, traditional established businesses will take a decade to adopt the AI available to them today. Yes, there will be competitive pressure for existing businesses to adopt AI, but they simply don't move quickly. Yes, AI will sneak in through ERP systems, Microsoft CoPilot, etc. But many average businesses struggle to keep their ERP systems up to date and supported, let alone do the digital transformation required to redesign processes to embrace all AI can offer them.
Costs, or the moment, it seems model costs are decreasing year on year in terms of bang for your buck. Look at the figures in this article just published,Welcome to LLMflation – LLM inference cost is going down fast, here
For an LLM of equivalent performance, the cost is decreasing by 10x every year.
The cost of LLM inference has dropped by a factor of 1,000 in 3 years.
Not only has the cost of inference dropped, this post points to the huge leaps that have been made in a short time. The AI Inference Revolution: Faster, Cheaper, and More Disruptive Than We Thought , a quote that post below...
Cerebras has shattered expectations, delivering inference speeds up to 20 times faster than GPU-based solutions at a fraction of the cost. The AI revolution isn't coming – it's already here, and it's accelerating faster than anyone imagined.
Now I've not verified the figures but I have no doubt it is not far from the truth. Due to the factors they list:
Better cost/performance of the GPUs.
Model quantization.
Software optimizations
Smaller models.
Better instruction tuning.
Open source contributing to drive.
These reducing costs make AI operating costs something that smaller businesses, can absorb. In SMB-sized businesses, efficiencies will come through cost workforce savings, more efficiency in processes and accuracy of work completed. AI applied well, can foster product innovation and more. Those may also contribute to the improved sustainability of businesses, but also provides some big disruptor tools for new companies looking to insert themselves into existing market spaces.
The Limits
However, technology tends to follow an S curve rather than an exponential curve. There has to be a point where the business side of the industry struggles to reach further performance improvements.
This very long article, The AI Revolution: The Road to Superintelligence, is a great read and is repeatedly referenced in various places in AI spaces. It breaks down the growth of AI into many S curves, which is what is experienced on the ground (see the little guy on the pic), even if the overall effect is an exponential curve.
Summary
Right now, it seems like things are moving at such a pace, but the problems to solve, to improve performance, are getting increasingly complex. To me, it feels like we are on the rise of another S curve, but with some "flatter" times ahead as the industry grapples with scaling, energy, capacity, and finally adoption.
I've explored that general metrics and vibe for the pace of progress in AI are not the same as those of us working in enterprise AI solutions. Those settings are more tangible, rooted in cost benefit to business, percentage adoption by business, rather than model sizes and raw performance etc.
These are indeed exciting times in AI and with these big minds solving the next set of problem, it could well be AI is set to grow quickly for some time ahead in the business context.
Its positive to see universities getting access to the resources they require to do good foundational research, something it looks like they were increasingly cut out of due to resource constraints before.
This basic overview I hope helped introduce some of the concepts and terminology that you will encounter when getting started with AI. Is is part of a series of posts on AI concepts.
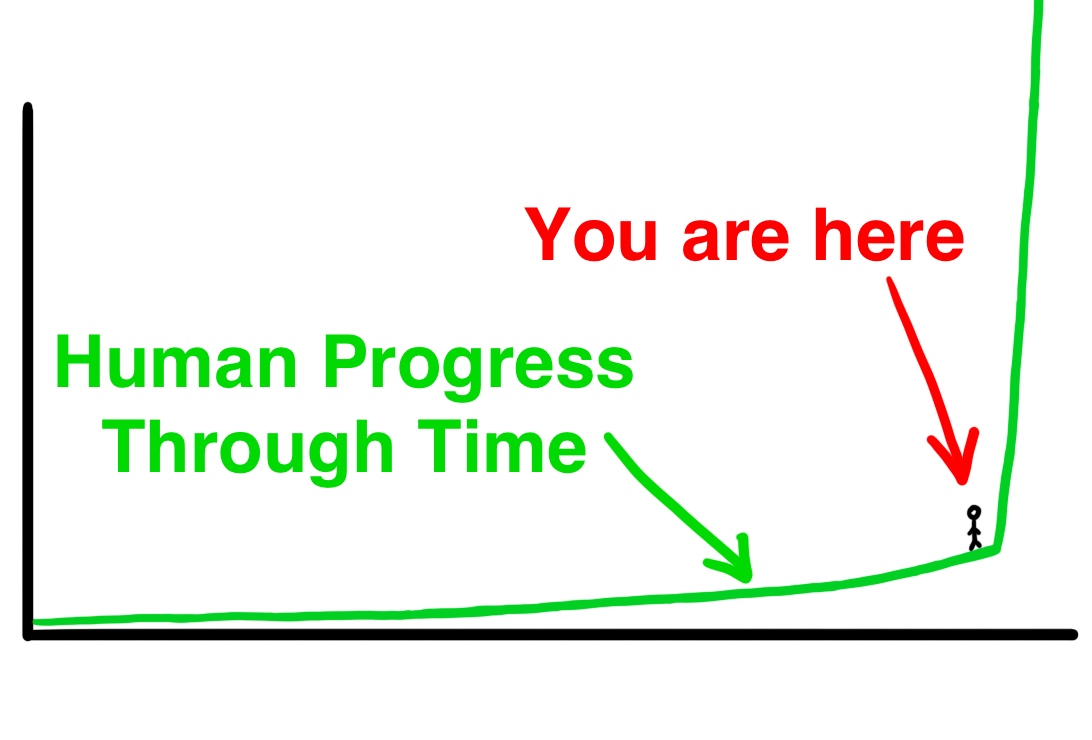
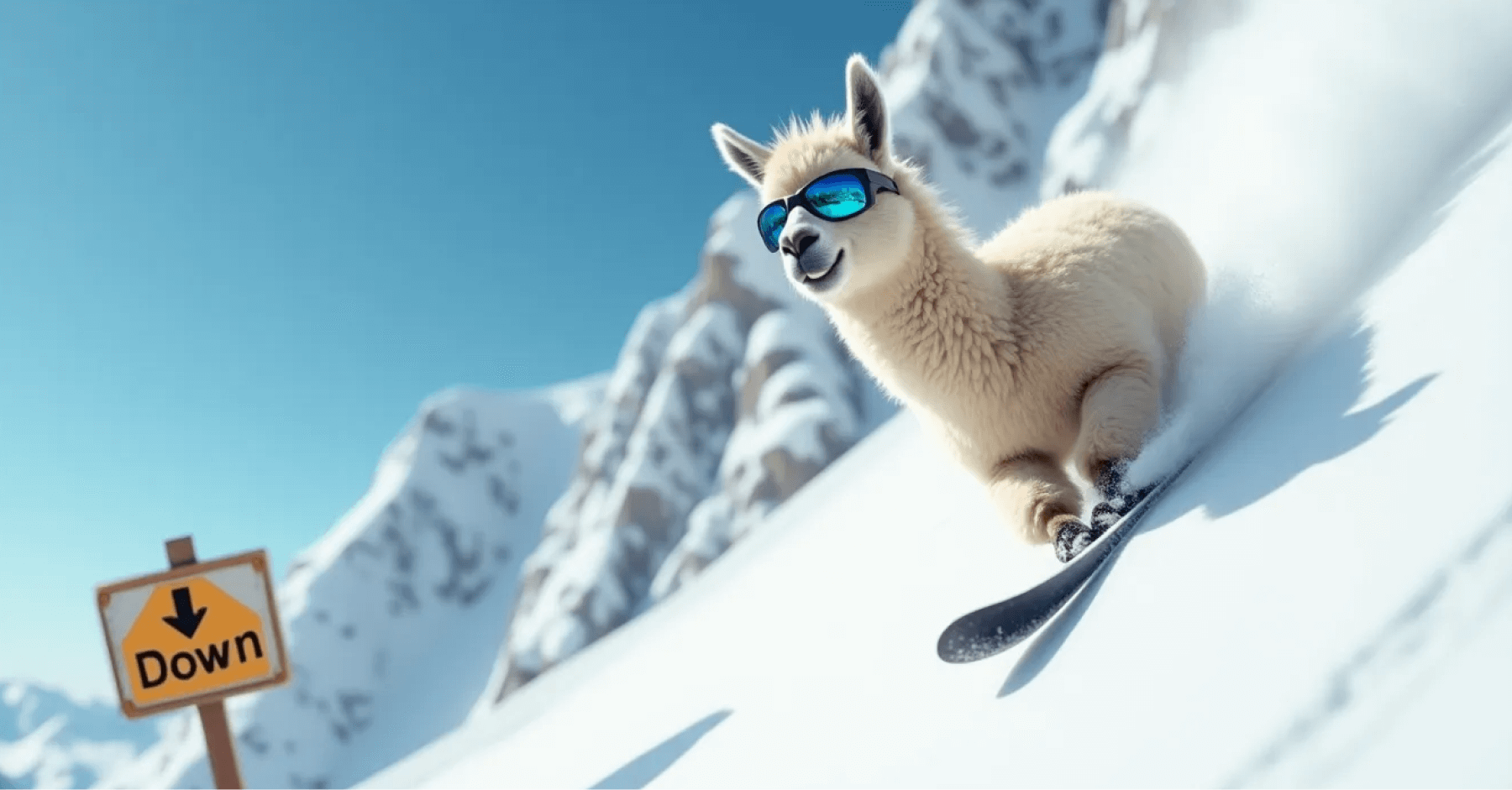