Paradigm shift from Search engines to Answer engines & Perplexity
Is traditional search dead, users are trying answer engines that give great results but with increasing toll on the environment.
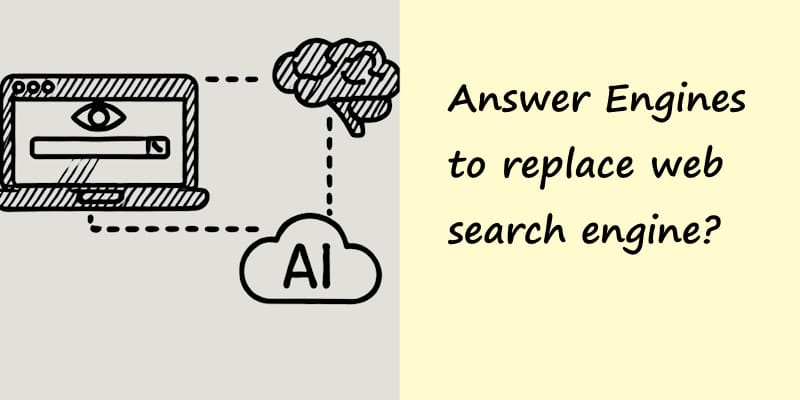
If you have not already, I urge you to go and explore Perplexity. I know a lot of people have significantly reduced their use of Google Search since exploring Perplexity. Indeed, some have stopped using Google Search entirely. This shift in adoption away from the pervasive market leader in search is significant. Take notice—go and explore the paradigm shift that Perplexity demonstrates, moving from a search engine to an answer engine.
Admittedly, your mileage may vary depending on how you consume search and ChatGPT, as well as the type of user you are. Developers find it easy and rewarding to make the switch, but rest assured, the masses will be using answer engines before long.
What is it?
Perplexity AI is a free-to-use (with usage limits for free users) AI-powered search engine that uses natural language processing (NLP) and machine learning to provide answers to user queries. It delivers a single, comprehensive answer that summarises everything you need to know. Perplexity searches the web in real time to ensure the information is up-to-date and current. It also integrates with data sources in real time, avoiding the need for users to leave the platform. Citations for results are provided, and it often offers follow-up suggestion searches alongside its answers.
It’s like having your LLM, Claude, or ChatGPT combined with web search, enabling users to ask questions and receive summarised, up-to-date answers. This is a vast improvement over the out-of-date information captured in static models and the lack of summarisation in traditional search engines. Whist recently ChatGPT has added the ability to reach out to the web, it feels like it is more of a first class feature in Perplexity.
It can lie!
Now, let’s get this out of the way—be prepared for wrong answers. Perplexity, when it doesn't have enough data to answer, will brazenly lie through hallucination. This is a challenge faced by all large language model (LLM) AI solutions. AI is often too eager to please. However, with this understanding in mind, it remains a very helpful and efficient tool.
More about it
There are two kinds of searches:
Quick Search
Quick Search provides fast but basic answers. It delivers immediate responses by quickly summarising relevant information from indexed sources.
Pro Search
If you’ve read my blog post on Logic of Thought prompting, you’ll understand the difference between rapid responses and Logic of Thought prompting. Under the hood, Pro Search employs LoT and is more costly to serve. It delves deeper into your question and may ask follow-up questions to refine the search, delivering more tailored responses. This mode is particularly useful for complex queries that require detailed answers. Since it consumes much more power and energy, only a limited number of queries are included for free (at the time of writing, five for free, or 600 per day with a paid plan).
You can perform much more complex reasoning searches than is typically possible with Google Search, although Google has a larger and fresher base index of web content compared to Perplexity.
Context
Perplexity uses context, so it will remember your session, allowing follow-up questions to be asked seamlessly.
Information sources
Perplexity strives for transparency. References to the sources of information are included in the output, often in footnotes.
Learning Spaces, Focus, and Rewrite will transform how you work. Each feature is explained below:
Spaces (previously called collections)
You can save similar searches into Spaces (workspaces). This makes it easier to group work together and collaborate. Search files and threads are kept together in a Space, keeping similar topics organised.
The real power comes with* combining custom system prompts with Spaces*.
Try out Focus and Rewrite
- Rewrite allows you to re-run your query using a different model. This lets you easily compare differences in output between various AI models (available to Pro users only).
- Focus offers modes like Reddit, Academic, and YouTube. Choose a mode based on the nature of your search to get the most relevant results.
Prompt to search
Searches are performed by writing prompts. It may be worth reading my introduction to prompting blog post to learn how to craft effective prompts. The better crafted the prompt, the better your experience will be. Rather than using brief questions, more structured, verbose queries yield better results.
Environmental impact and future
After encouraging you to try Perplexity, I now ask you to consider the environment. Mass adoption of AI for search will have significant environmental impacts. AI requires substantial computational resources, which translates to high energy consumption. LLM inference and APIs are far more energy-intensive than traditional search.
Perplexity must currently be burning through investor money at a rapid pace. They have not yet had to contend with the search volumes Google handles daily, and it will be interesting to see how financially sustainable this model is. Can they demonstrate enough value to justify users paying high subscription fees to sustain the service? While more efficient methods of serving AI are continually being developed, it’s unlikely they will provide the drastic improvements required for AI search to scale in an environmentally friendly way.
Lets try something Google search couldn't do...
The website Ollama library (screen shot below), has a list of AI models that I would like to extract and reformat as a table, adding in information not on that web page. I want the extracted list in extended markup for my blog. As I want to know information on each model that is not on that page, who makes each model, so I need this looking up for each item.
The format of the list I want to format and augment,
So I write a prompt, using normal prompting techniques to explain the problem and the format example, I end up with this...
Perplexity query
Go to the page https://ollama.com/library
Make a list of all the models on that page. Look up each model to find the company behind it. If no company do not list it.
Use the format
Name, company, model type
use advanced mark down to put this into a three column table in markdown format I can paste
On my first go I get a results set just how I wanted it, super time saver and less error prone than lots of copy and paste and manual look ups.
Result
Update 2024-11-28
Podcast
This podcast is well worth a listen to: The TED AI Show, in it Perplexity CEO Aravind Srinivas does a good job of describing how Perplexity are positioning themselves, what the financial model looks like. The plans go much deeper than what you see today.